Blog
Statistics for Sustainable Development > Blog > 6 Principles for Data Visualisation
6 Principles for Data Visualisation
Data
visualisations are everywhere. It seems they are becoming more prevalent in our
day to day lives, and not just for us as statisticians, but for everyone. This
seems especially true in the days of COVID-19. For months we could not go a day
without seeing the latest graphs on cases, hospital admissions, and deaths being
shown at the daily briefings. These graphs continue to dominate news coverage.
Data visualisations are arguably the best way to communicate clear messages to the public. But for these messages to be clearly understood and avoid misinterpretation, they need to be well designed and chosen carefully. Poor decisions in graphing data can result easily in wildly different and misleading messages. My mind thinks back to the infamous plotting of the Florida gun deaths from a few years ago, which inexplicably put the 0 at the top of the y-axis, making at first glance the increases look like decreases and vice versa.
Image 1. Famously misleading plot of gun deaths in Florida
To help everyone make better data visualisations, I have drawn up six guiding principles which should help in getting started. These are relevant whether you are making a table, graph, or map.
1. Effectiveness
Effectiveness
concerns arguably the most important step - choosing the right type of
visualisation. The visualisation you choose for the data and its message, can
make a big difference to its effectiveness. For example, trying to plot
anything other than proportions that total 100% onto a pie/donut chart just does
not work.
A general
guide would be that maps are for spatial data, tables are suited for structured
numerical information and graphs are very multi-purpose - but are primarily
used for indicating trends, making broad comparisons, and showing
relationships. Tables can be used for similar purposes, the difference being
the specific numbers are more important to a table while the broad patterns are
the focus of a graph.
If you are
choosing a graph, you need to then consider what type of graph to use. This is far
too broad a consideration and could be multiple blog posts in of itself. Therefore,
for more advice, please look at our resource on Presentation of Tables, Graphs and Maps.
For
example, the pie chart below, while it looks pretty, it has too many categories
and therefore it becomes increasingly difficult to read the differences between
groups. Plus, it is incredibly difficult to get at any actual proportions
because it is all based upon area. Moreover, for this type of data it is not
really the proportions that matter. It is the numbers. This graph would have
been much more effective as a table.
Image 2. Unnecessary pie chart of national contributions to the sample size of European Social Survey (ESS) 2018
2. Informative
Making sure
your graphs are as informative as possible serves two purposes. Firstly, any
data visualisation needs a clear message and a clear need. It must have a
reason to exist. If there is no apparent message to the data, then the visualisation
becomes just a bunch of white noise with nothing to say. It fails to inform because
it has no useful information.
Once we
have created a data visualisation and it has been publicly shared, it can be
difficult to control exactly how people choose to share, present or even
recontextualise. Realistically we should be able to provide/withdraw consent to
certain uses of our visualisations, but we can’t keep track of every usage.
This is the
second purpose of being informative - providing enough information as possible
to ensure that our visualisations can be understood when taken out of the
context of its original publication. Any good visualisation should be able to
be understood outside its original use and be able to serve as a stand-alone
piece.
Therefore, be sure to provide source notes where required as this aids credibility. Additionally, you should be including relevant details such as measurement units, dates, analysis units, maybe footnotes to explain acronyms.
3. Readability
Readability
is quite a simple concept that hopefully you should already be following. All
elements of your visualisation should be legible, understandable, and coherent.
This largely concerns the text elements of the graph such as titles, labels,
notes etc.
Titles and
headings should concisely explain the content and should not be needlessly long
and complicated. The same goes for any axis markers, labels etc.
Of course, the elements will depend on the type of visualisation, but they should always be easy to read and understand. Avoid using language beyond the scope of your target audience. Using relatively broad language will also aid in making your visualisation informative to everyone not just your originally intended audience.
4. Tidiness
This is a
similar idea to readability but focuses more on the positioning and spacing of
elements and avoiding unnecessary clutter. The purpose is the same; making sure
your visualisation can be read and understood.
This
includes making sure that no elements are overlapping, there should be adequate
spacing between them. Although not too much, otherwise the visualisation will
just look empty. Make good use of the “white space”.
This also
includes trying to avoid overplotting. Overplotting is when data points overlap
making it difficult to read different points. Usually this is because there are
too many data points with the same/similar values, or there are a limited
number of unique values. This can be avoided by reducing the size of points,
sub-setting the data, using transparent symbols, or jittering the points. See
more examples in the resource guide. In the graphs below, there are few points
because so many are lying on top of each other making the plot look quite empty.
The second graph shows how this overplotting can be fixed with a little
jittering of the points.
Also, tidiness means avoiding using “junk” features. E.g., shaded backgrounds, borders, patterns, textures, shadows, 3-D graphics etc. Anything that provides no real purpose other than to take up space.
Image 3. An example of overplotting
Image 4. Fixed overplotting by jittering the points
5. Consistency
This is
largely relevant if you are intending to use multiple visualisations. It is
important to maintain a level of internal consistency.
This
involves many aspects, but I think there are two broad ideas at play. Firstly,
be consistent with how you plot the data on your visualisation. This means
paying attention to points such as order of categories. The order should be
kept to a logical or ascending/descending order of a variable. Unless ranking
is important to your message, try to keep the order the same throughout. Similarly,
if you assign colours to categories, keep these colour schemes the same
throughout. Do not change them up at random points as this is just confusing.
Secondly, there should be some level of “design” consistency. So, pay attention to keeping the finer details the same. These are things such as the font, size, face etc. Try to keep the formatting as consistent as possible.
6. Accessibility
Finally,
accessibility is increasingly important to data visualisation. We want to make
sure as many people as possible will be able to look at our visualisation and
understand its message. This includes using non-technical language as mentioned
previously, but also considers how to make the content accessible to those with
impairments, especially those with difficulties with their vision.
For data
visualisations, a lot of this comes down to accounting for colour blindness[1].
This affects about 1 in 12 men and 1 in 200 women. Most commonly “red-green” vision
deficiency. Therefore, if we use colours which are inaccessible to people with
these impairments, they will not be able to properly read our visualisations
and leave them alienated from our research. The two graphs below show just how
different someone else may see our graphs.
There are endless more specific tips and tricks that I could
detail here but many are largely dependent on your data, your messages, and the
types of visualisations you choose to create. However, adhering to these 6
general principles of effectiveness, being informative, readability, tidiness, consistency,
and accessibility, will hopefully help in getting started and enable you to
think a bit more carefully when designing your own data visualisations.
Sources:
Image 1: Gun
death graph: https://www.livescience.com/45083-misleading-gun-death-chart.html
Image 2: Data
for Pie Chart: European Social Survey 2018
Images 3 and 4: Exam Failure Graphs: Simulated data
Image 5: Colour blindness example: Created by Emily Nevitt (Stats4SD)
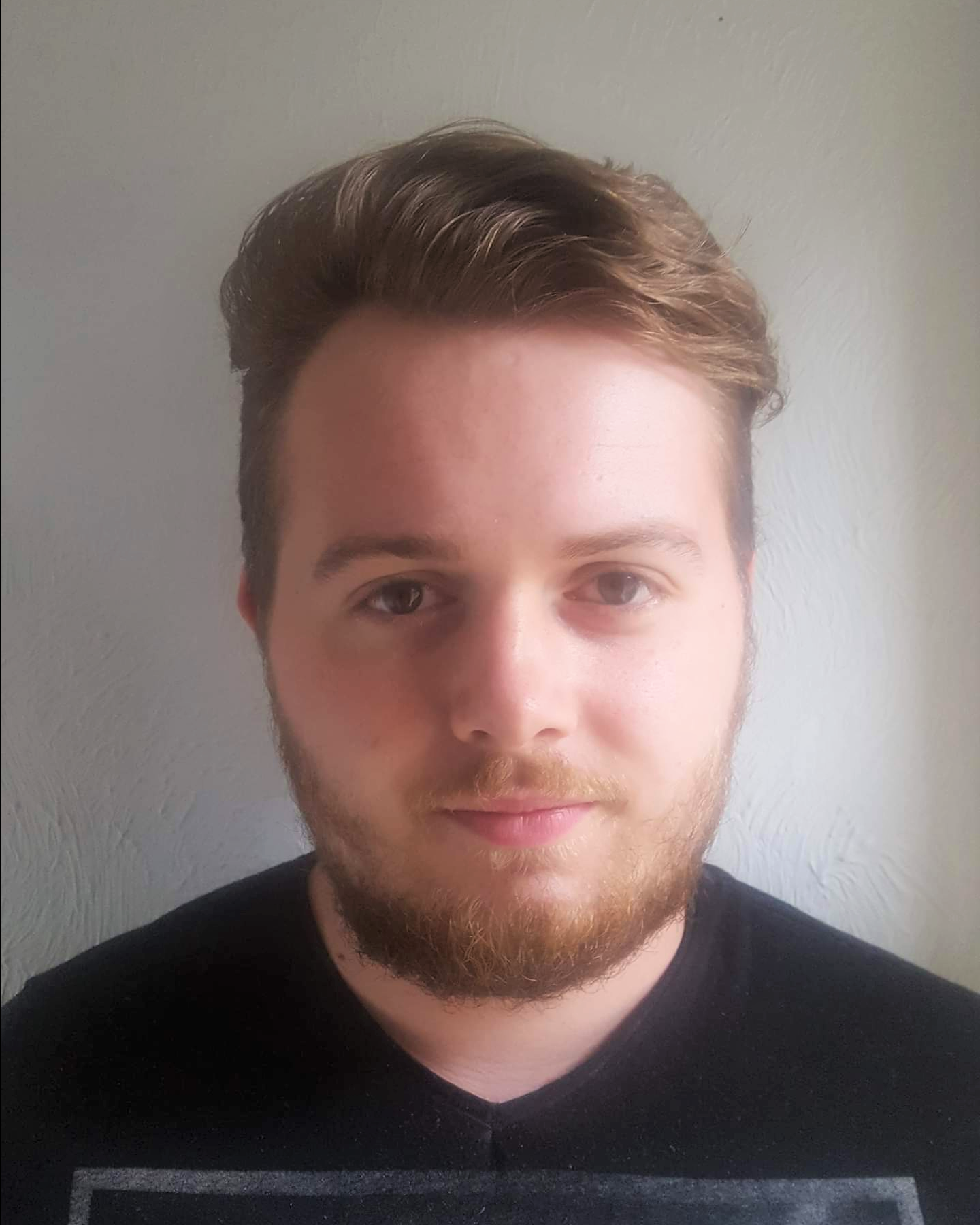
Author: Alex Thomson
Alex joined the team as a Statistics Intern in October 2019 following the completion of his Undergraduate and Master’s degrees in Population and Geography, and Social Research Methods at the University of Southampton. With a background in demographic and social science research, especially family demography - Alex hopes to extend both his skillset and knowledge base of issues affecting the developing world and building upon an undergraduate trip to Ghana. With experience in STATA and SPSS, Alex hopes to develop his skills in R, survey design and management while at Stats4SD.
0 comments for "6 Principles for Data Visualisation":
Add a comment:
We run an anonymous commenting system. If you are not logged in, we do not collect any information on who you are when you leave a comment. This means we manually confirm comments before they appear on the site.
If you want to have a comment you submitted deleted, please contact us, giving the date of the comment and name of the article.