Blog
Statistics for Sustainable Development > Blog > Beyond academia: lessons from a statistical intern
Beyond academia: lessons from a statistical intern
Introduction
At the core of any statistician is somebody who enjoys solving problems and I'm no exception. I really enjoyed my time at university, but the courses emphasised abstract and theoretical problem solving, and after a while I began craving seeing real applications of statistics - away from the curated data sets and the ideal assumptions. As well as this, I would gravitate towards problems that involved social aspects, hoping to find work that balanced the challenging abstract thinking with the complexities of social behaviour. With what I just said, you can imagine how pleased I was to find Stats4SD after leaving my masters programme!
Five months after joining the organisation, I have completed my first international trip to Ethiopia, and have been asked to write up my thoughts. I went with my colleague Sam to support postgraduate students build their statistical and R capacity. By chance, our trip coincided with the ‘Hawassa Math & Stat Conference 2019’, so as part of my career development training fund, provided as part of my internship, Sam and I attended. There are more things I learnt on the trip that I can fit into this blog, so I will focus on a few lessons I learnt along the way.
Teaching ggplot2 to students
There is no ‘best’ model
One concern I had during my masters in statistics was not learning the ‘right’ techniques. With the growing influence of data science as a paradigm for data analysis, all I could read about was the incredible potential value that neural networks and related algorithms offered. These feelings motivated me to leave academia and see how organisations working with real problems apply these techniques. Stats4SD is filled with talented people working with challenging problems, and I knew through joining the team I would find my answers. During my time, each time questions about modelling arose, the same conclusion was reached; "What is your question?". My trip to Hawassa really consolidated this idea.
Models are rarely built for their own sake but are built to help answer questions; be it an agronomist trying to determine optimal seed variety, or an investor trying to minimise risk in their portfolio. The conference provided a breadth of examples of different applications, where each model offered a way of framing the original research question based on the available data and information. The more models you know, the more perspectives you can approach in your analysis. There really isn't a ‘best’ model - the value of any modelling approach is instead determined by its ability to answer the motivating questions.
Statistical analysis is a decision problem
Many factors go into determining how to approach a problem; dataset and available expert information jump to my mind, but contextual factors such as available time and financial resources also play a major role in this and the idea of a ‘best’ model becomes even more ambiguous. Granted, a model produced at the end of a three-year research project would likely provide more accurate predictions, however these may not change decisions - and at what cost? At Hawassa, one of the talks directly addressed this point. A student of the speaker had been placed modelling an industrial pasteurisation process. By the time the project was completed, the results confirmed the current process was appropriate - nothing needs to change! There is huge value in being more certain about decisions, but it was an interesting insight for me - simpler models can provide appropriate decisions. For me, effective data analysis is thinking about the question, and deciding on appropriate actions and clarifying before you proceed is useful.
Assumptions are unavoidable
With the best will in the world, you cannot be certain about your model assumptions. If you are fortunate, you can draw upon a wealth of knowledge from papers and peers to build confidence in a certain approach, but even in these circumstances, you will have to draw a line and include information in your model that you are uncertain about - and that's okay.
A very common model is the two sample unpaired t-test. The t-test theoretically requires your population to be normal [Note:Test]. That said, practically speaking, your never know if your population distribution is normal, and if you did, you probably wouldn't need the test in the first place. So should we abandon the t-test?
Well, as a famous quote goes, "all models are wrong, but some are useful". It highlights that all modelling is built upon ‘untrue’ assumptions, so the real question when selecting a model isn't about whether your assumptions are ‘true’ or ‘false’, it's about ‘how’ wrong they are, and are there any decision-changing consequences as a result? In the case of the t-test, my question becomes: is my population distribution close enough to a normal distribution? Simulations have shown [Note:Sim] that the decisions based on results of the t-test are fairly robust to moderately non-normal population distributions. Again, the emphasis isn't on how ‘true’ the model is in a binary sense, rather whether the non-normality would affect any conclusions drawn. Making informed decisions about what is appropriate is what makes a good statistician, and those judgement calls are one part of what make a statistical consultant useful.
Since I have used a lot of quotes in this section, it seems appropriate to end with one too:
"To consult the statistician after an experiment is finished is often merely to ask him to conduct a post mortem examination. He can perhaps say what the experiment died of."
- Ronald Fisher
Conclusions
I'm only at the beginning of my journey as a statistician, and my ideas will inevitably evolve, but these points feel fundamental. I can now understand some of the nuances in model selection, how to balance theoretical with practical demands and how to ensure my analysis meets the demand. These lessons are only very much appreciated by me because I have been given the opportunities and guidance by Stats4SD to explore them in my own way. I hope by sharing these ideas, it can help other early-career statisticians.
References
[Note:Sim] - https://www.annualreviews.org/doi/pdf/10.1146/annurev.publhealth.23.100901.140546 [Note:Test] - for those interested, otherwise the distribution of your sample variance does not converge to a chi-square. See https://thestatsgeek.com/2013/09/28/the-t-test-and-robustness-to-non-normality/
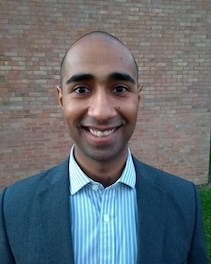
Author: Faheem Ashraf
Faheem joined the team in October 2018 as a Statistics Intern. He is driven by a deep interest in reconciling the gaps between the academic statistics taught in his Mathematics Bachelors and Statistics Masters with the demands of real-world data analysis. Faheem intends to use his time at Stats4SD to explore the breadth of socially pertinent problems that can, and cannot, be meaningfully approached using Statistics. With experience in SAS, Excel, Python and especially R for data analysis, combined with his friendly personality, Faheem hopes to use these skills to present his analysis in an approachable and insightful way.
0 comments for "Beyond academia: lessons from a statistical intern":
Add a comment:
We run an anonymous commenting system. If you are not logged in, we do not collect any information on who you are when you leave a comment. This means we manually confirm comments before they appear on the site.
If you want to have a comment you submitted deleted, please contact us, giving the date of the comment and name of the article.