Blog
Statistics for Sustainable Development > Blog > On sampling and randomness pulled out of the hat
On sampling and randomness pulled out of the hat
“The purely random sample is the only kind
that can be examined with confidence by means of statistical theory, but there
is one thing wrong with it. It is so difficult and expensive to obtain for many
uses that sheer cost eliminates it. A more economical substitute, which is
almost universally used in such fields as opinion polling and market research,
is called stratified random sampling.”
Darrell Huff, How to Lie with Statistics
Why
Sampling?
Decision makers constantly need information on characteristics of a population. Due to timeliness and cost reasons, this information is often obtained through sample surveys. The process of selecting a part of the population to observe, so one can estimate its characteristics of interest, is called sampling.
However, while the population
characteristics remain fixed, its estimate depends on which sample is selected.
With careful attention to the sampling design and using a suitable estimation
method, one can obtain estimates that are unbiased for population quantities.
The estimate is unbiased if its expected value, over all possible samples that
might be selected with the design, equals the actual population value[1].
The
need for random selection
In order to remove known and unknown
human sources of bias, such as conscious or unconscious tendencies to help select
units with specific characteristics, a probability selection of samples is
needed. A probability design such as simple random sampling can therefore
provide unbiased estimates of the population characteristics, such as the mean,
total or a proportion. Simple random sampling is the sampling scheme in which
units are selected in such a way that each unit has exactly the same
probability of being chosen. Unbiased estimates can also be obtained from unequal
probability designs; provided the probability
of inclusion in the sample is known for each unit. Both types of designs allow
for the estimate of the variability and the computation of the estimate’s
precision.
Sampling
Strategies
Simple random sampling can be
expensive and often not feasible, since it requires that all elements of the
population are identified and labelled prior to the sampling (in survey
sampling these lists of units are called sampling frames). And this is often
not possible. Also, it implies that each unit has an equal chance of being
selected, and this may result in a sample that is spread out over a large
geographical area, which would be very costly to implement[2].
Though in practice one may never decide
to select a design based on simple random sampling, it is the simplest possible
method, and therefore the one against which all other methods can be compared
against. Eventually, even in more complicated designs, there might be a stage
in which units are selected by simple random sampling.
An alternative is to use a
sampling plan that involves different stages. The simplest one involves two
stages. In the first one, the population is divided into clusters and a random
selection of clusters is obtained. In the second stage, a random sample is
drawn from each of the clusters. In such a scheme, sampling units have
different probabilities to be selected, but these probabilities can be computed
if the two stages are carried out wisely.
So, in the first stage, the
sampling units are the clusters, and a comprehensive list of these is much
easier to obtain. There are different approaches on how to select these
clusters from the list, but all of them involve some degree of randomness
(including a simple random sample of clusters). In the second stage, the
sampling frame will include all the units within the selected clusters, and again
a random process is used to select units within clusters, usually simple random
sampling.
A
case study: random sampling in the Pamir Highlands
The simplest way to understand simple
random sampling is to think of someone pulling slips of paper at random out of
a hat. Every slip of paper in the hat has an equal chance of being plucked out.
So, if every slip of paper has a name on it, every name has an equal chance of
getting picked, meaning that it is “random” which names get picked.
Picking slips of paper from a hat
to select a simple random sample is a very powerful image, but it is never used
in practice. Who wears a hat today? Never say never! In a survey carried out in
the Gorno Badakhshan region in Tajikistan, in the Pamir highlands, a 2-stage
sampling design was chosen. The population was split into administrative
regions (districts), and data from all districts was collected. This process is
called stratification, and its goal is to ensure that data from all districts
will be collected. The first stage was the random selection of clusters. A
cluster in this case is a village, except for the cases of larger villages that
were split into clusters of similar size. Finally, a simple random sample of
households was selected for interview within the selected clusters, using
simple random sampling.
In smaller villages, heads of the village have a comprehensive list of the households in the village, so the process of selecting a sample was done using a hat and slips of paper!! The team of enumerators, belonging to the Aga Khan Agency for Habitat – AKAH, took pictures of the process. It was not always an easy process, though! In such welcoming areas, more than one head of the village insisted that they would be very delighted if the team could start the interviews from their own house, regardless if it was selected through the random process! An additional bit of explanation and some apologies were required to sort this out!
Fig. 1: the AKAH team supervisor is holding a traditional hat with the paper slips
Fig. 2: random selection of a
sample by plucking slips of paper from the traditional hat
Fig. 3: random selection of a
sample by plucking slips of paper from a hat in a Pamiri house
References
[1] Thompson, S.K.
(1992) Sampling. New York: Wiley
[2] Levy, P. S.; Lemeshow, S. (1999) Sampling of Populations. New York: Wiley
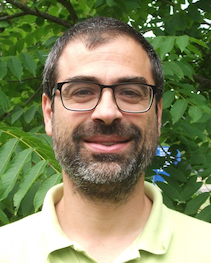
Author: Alex Riba (old)
Alex is an engineer with over 20 years of experience teaching statistics and conducting research. Having worked as a statistician on a wide range of projects, he is particularly interested in processes that let data speak for itself, especially in meaningful ways for non-statisticians.
0 comments for "On sampling and randomness pulled out of the hat":
Add a comment:
We run an anonymous commenting system. If you are not logged in, we do not collect any information on who you are when you leave a comment. This means we manually confirm comments before they appear on the site.
If you want to have a comment you submitted deleted, please contact us, giving the date of the comment and name of the article.