Blog
Statistics for Sustainable Development > Blog > Improve your ODK forms in 6 easy steps
Improve your ODK forms in 6 easy steps
If you only have 5 minutes and want to skip straight to the ODK tips – click here. Otherwise, read on…
Preamble – The Dark Ages of Digital Data Collection
In the past, doing 'digital' data collection involved a months-long game of telephone, where the Principal Investigator (PI) would tell the data manager to write a form, then they'd tell the programmer to make a digital version, who'd show it to the Data Manager, who'd show it to the PI, who'd request a bunch of changes, and so on. Then, (if they were following good practice), they'd run a pilot test and find a whole bunch more to change, and the cycle would continue.
The result would be a bespoke data collection tool that only the programmer knew how to update, only the data manager knew how to use and only the PI knew the purpose of. The tool would be used once - maybe twice, then never looked at again.
It's no surprise that most data were collected on pieces of paper!
Our Saviour…
Fortunately, we don't live in such dark times any more. Smartphones and the internet have brought with them a variety of powerful ways to collect data without having to code an application from scratch. For us, the big player here is the Open Data Kit - powerful, flexible, open source - a tool dedicated to making it as easy as possible to collect survey and experiment with data using cheap mobile phones.
One of the most powerful things about ODK is that you don't need programmers to come and build your forms for you. Any researcher with decent spreadsheet skills can build a form using the XLS-Form standard, upload it to a service like Kobotoolbox and have it on their phone ready to collect data within an hour of deciding to run a survey. Bad news for the programmer who’s suddenly out of a job - but is it good news for the researcher? On balance, yes, but such power always comes with a cost.
…Our downfall?
ODK makes it easy to collect data. But, it also makes it easy to collect terrible data.
The tools have removed so many of the barriers between idea and implementation, but by being so flexible they allow us to get away with ignoring a lot of ‘good practice’ around data collection. It's easy to forget the rigour and careful planning that's required to design a truly good data collection form. A good form asks “the right questions, at the right time, to the right people” – and doing all 3 of those is surprisingly hard!
So how do we address this problem? How do we keep using these quick and flexible tools without falling into the trap of collecting too much data of unknown quality – just because we can?
ODK – 'Quick and Easy' data collection
We often sell ODK to new users with the promise of speed and simplicity. It’s quick to get started - you just need 3 columns and you immediately have a valid, working form. Take this example.
This form is perfectly functional. If you save this in Excel and upload it to Kobotoolbox, you can immediately start asking people about their caffeine habits. Great! Quick and easy, as promised.
Now let's take a look at some data collected with this form:
Quick challenge – spend 1 minute looking at the data above. How many issues can you see in these records? Write them down, then read on to see how many you found.
Data Quality Issues:
- Gender is written in many different ways, making it hard to group by gender.
- There are missing values in all 4 data columns. There could be many reasons:
- The enumerator made a mistake in entering the data.
- The respondent didn’t want to give a response.
- In row 3, the missing values could be because the respondent does not drink caffeinated drinks, but we have no way of knowing for sure.
- In row 2: 245 is not a valid age.
- The ‘caffeine_yn’ column should be just ‘yes’ or ‘no’, but like ‘gender’, it has lots of variations.
- ‘Cofee’ in Row 1 is misspelt.
- Row 2 has conflicting answers: the respondent answered ‘no’ to whether they drink caffeinated drinks, but then said they drink Red Bull…
- “I drink red bull” – a full sentence in this context is going to be a pain to analyse via quantitative methods.
- 2 people listed multiple drinks, but they’re formatted differently – Row 4 has a comma separated list and row 6 has a space-separated list.
Now, obviously this example is faked to show off lots of issues. But I have seen every single issue here in real data collected with ODK, usually at the point where it’s too late to go back and check with the enumerators. So what can we do about it?
Fortunately, all of these issues are preventable by using existing features of ODK. While type, name and label are the only ‘essential’ columns needed for a functioning ODK form, there are some features that are just as vital for collecting good quality data.
ODK – 'Smart' data collection
If you only have 5 minutes - start reading here!
So, you want to improve your ODK forms. You have some basic questions written as an XLS form, and you want to add some quality control. Where do you start?
One of the key things to remember is that humans are fallible. We all make mistakes, and we make lots of them during easy, mundane activities like data entry. You may assume that no-one will accidentally enter 245 instead of 24 into the 'Age' field, but after 6 years of doing data quality checks, I can assure you this type of mistake is not only likely; it is almost guaranteed, even in surveys of just few hundred records.
Fortunately, ODK has a lot of useful features you can use to limit these sorts of mistake. The tips below are all quick to add to a form; not too technical and will dramatically improve the quality of your data:
1. Use the constraints column:
- Every number needs a constraint.
- For age, you should prevent entries less than 0 and above a sensible maximum. I normally use 0 <= . < 150 that limits responses to numbers between 0 and 149 inclusive.
- Add a message using the constraint_message column. This gives enumerators information about the constraint when they enter an invalid answer.
2. Make questions required:
- This prevents the problem of ambiguous missing values.
- To cover all possibilities, you should provide an option to say "the respondent didn't answer". For numeric questions, this could be a code, like "-99". for a non-response to an age question. For select questions, add an option for 'no response' - and make sure the enumerator knows not to read this option out!
3. Only show relevant questions using the relevant column:
- This prevents many situations where conflicting or incompatible responses can be given.
- It also allows 'optional' questions to be marked as required to avoid blanks. A question will only be required if it's actually relevant.
4. Always use select questions instead of text when possible.
- In the example above, if gender was a select_one question, most of the issues with the gender data would have been avoided.
- Similarly, many problems with the caffeine question could be avoided by using a select_multiple instead of text
- Make good use of the 'or_other' feature for select questions. This allows responses you didn't think of ahead of time - and those responses are often where the most interesting and surprising results come from!
5. Good, clear labels to questions are good. Good, informative hints are even better!
- Use hints to give your enumerators extra prompts for questions, to remind them about specific wording or remind them of specific parts of the training.
- For interviews, I usually suggest making the label only what the enumerator should read out, and put any instructions to the enumerators in the hint. The easier you make life for the enumerators, the more reliable your data will be.
The last tip here is a bit more complex than the others. I'm including it because it answers a very common challenge - how to filter through a set of nested options lists, for example how to identify a specific household.
6. For filtering long lists, use a series of select questions and choice filters.
- This lets you filter a very long list of options using the value of a previous question. For example:
- You have a list of households, split by community and then by district.
- Instead of just presenting the huge list of households, you can instead ask 'which district?' Then, you ask, 'which community?' and present the list of communities within that district. Finally, you have a manageable list of households from within a single community to choose from.
So, using these tips, what would our caffeine form look like?
Worksheet: Survey
Worksheet: choices
I know - this looks a lot more complex than the first version of the form. We've added 5 new columns and an entirely new worksheet. It definitely takes longer to learn and write than the first version! But I hope you can see that it's not that much extra time, and I guarantee this updated form will give you data that is much more usable.
Even if you only do some of these - for example, making questions select_one or select_multiple instead of text, or adding some basic relevant code to improve your form flow, you will see a big difference in the quality of your collected data.
More Resources
If you want an annotated version of this example form, you can download the Excel files here. We also have an XLS form template available, which includes all the common column headings I’ve mentioned here.
For more discussion about the technical and non-technical aspects of writing good data collection forms, check out these videos.
Let us know in the comments if you found these tips useful – and if you have any neat ODK tips of your own. I’d like to do another ‘ODK Tips’ post in the future, so perhaps we can feature yours!
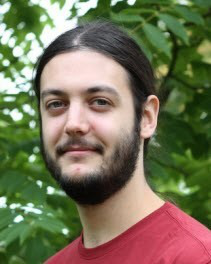
Author: Dave Mills
Dave developed an IT & data infrastructure that allows us to close information loops and deliver tailored information to diverse users, through data collecting mobile apps. He is also responsible for the development of our eLearning portfolio and Open Educational Resources.
2 comments for "Improve your ODK forms in 6 easy steps":
Gilbert
Nov 08 2021
Add a comment:
We run an anonymous commenting system. If you are not logged in, we do not collect any information on who you are when you leave a comment. This means we manually confirm comments before they appear on the site.
If you want to have a comment you submitted deleted, please contact us, giving the date of the comment and name of the article.
Jane
Sep 08 2019