Blog
Statistics for Sustainable Development > Blog > Using multiple knowledge sources to manage a mess
Using multiple knowledge sources to manage a mess
How do you deal with this? There is some 'quantity of interest' (QOI) that varies a lot between people and you want to know why. You have a lot of other data about each person that might provide some insights, and it's all nicely arranged in a spreadsheet with lots of columns and a row for each person. What next? If you learnt statistics as part of applied science, the answer would probably be some sort of multivariate modelling – multiple regression in one of its many guises. If you are of the Big Data generation then first thoughts will be of pattern discovery algorithms that you can run on the data. But in many of the cases we come across, neither of these are very helpful, at least on their own.
Last week I was working with a group of researchers in E Africa who are all working on soil health – research aimed at helping farmers improve and maintain the soil that is the basis for their livelihoods and the environment they depend on. Several of them have data from simple trials done by hundreds of farmers that compare alternative soil management strategies. The common feature of these is the huge variation in treatment effect across farms, so a key research question is "Why?". Each research team has ideas of possible reasons, collects the data and is immediately faced with the problem described – lots of variables that might be part of the answer, but few guides on what to do next.
Members of the CCRP Soil Health group in Eldoret, Kenya, discussing reasons why the performance of finger millet is so variable.
The regression approach does not work when there are too many variables – the models are simply scientifically unrealistic and the common statistical tools often of dubious validity with such real-world data. The data-driven approaches of Big Data have been developed for problems with millions of cases, not the few hundred we have. Our data sets take a lot of time, effort and dedication to generate and are large compared with those that appear in text books, but are very small compared to typical Big Data applications.
One way out of the mess is to structure the problem using other information. Statistical methods are often presented as if the aims, study design and nature of the data are enough to define the approach to use, but there is always more information and knowledge that can be used. In the soil health cases, the researchers have their basic soil science and the scientific literature relevant to the problem. They also have local knowledge from the farmers they work with, who typically have plenty of ideas as to what is causing variation even though they may express them in different terms than scientists. For example, scientists point out the very low organic matter in some soils which reduces its fertility and physical structure. Farmers call these soils 'dead'. There is often information from local experts who have studied related problems in the same area and there are researchers' own observations from the field.
We have found that a simple stepwise approach to using this information is very fruitful.
- Step one is to clearly identify the response or outcome that is the focus of analysis - the QOI.
- Next, we brainstorm to produce a list of all the factors that might be involved in explaining its variation. A long list of environmental, social and management factors that apply at various scales is quickly generated.
- Then organise this into the proximal factors expected to have direct causal influence and the more distal factors that operate through mediators. A box and arrow diagram helps to keep track.
- We then rationalise and simplify the diagram, removing those factors that, on reflection, are likely to have effects too small to detect or are outside the scope of the study.
- Finally do a reality-check: does the picture make scientific sense?
The resulting picture can now be used to structure the statistical analysis, its interpretation and reporting. It's not fixed and might well change as the analysis proceeds, but it provides a strong starting point.
"When I have tried this with groups like the soil health researchers and they always find it very insightful – a practical way from bewildering mess to manageable progress."
Applied statisticians tend to say something like "Is that all? I do that anyway." Maybe this approach is intuitive for experienced analysts who work in applied science. But it is not part of the good statistical practice that researchers learn and it should be. It helps make a close link between statistical method, scientific knowledge and local knowledge.
It also illustrates one way in which good applied statistics is an art. I have been criticised for saying that – statistics should be objective and art is inherently subjective. But two people drawing up influence diagrams will come up with different pictures, revealing their own interests and information. They could then come to different conclusions after analysis of the same set of data. Some find that disturbing, but it is the way real data analysis is.
Do you encounter these sorts of challenges in your work? Have you used similar methods to try and organise the mess into something more useful? Do you think this method is intuitive, or do you find it hard to know where to start? Let us know your thoughts in the comments below.
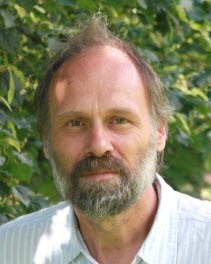
Author: Ric Coe
Ric’s main focus is on improving the quality and effectiveness of research for development using the application of statistical principles and ideas. He is particularly interested in research design, including the design of complex integrative research projects.
0 comments for "Using multiple knowledge sources to manage a mess":
Add a comment:
We run an anonymous commenting system. If you are not logged in, we do not collect any information on who you are when you leave a comment. This means we manually confirm comments before they appear on the site.
If you want to have a comment you submitted deleted, please contact us, giving the date of the comment and name of the article.