Blog
Statistics for Sustainable Development > Blog > Beyond ANOVA – Big Data and Large-N trials
Beyond ANOVA – Big Data and Large-N trials
We have been promoting and getting experience with using large-N trials with farmers. We have been reminded that 'large' is not always right and we should be thinking of the 'right-N trials’. But what do we mean by this, and how can the resulting data be analysed?
A typical large-N trial with farmers is one in which hundreds, or even thousands, of farmers compare alternatives by each doing a simple experiment on their own farm. In a previous blog, 'Using multiple knowledge sources to manage a mess’, I wrote about the different layers of data analysis from such a trial and the challenges of doing this. When researchers are trying to make sense of the aggregated data set, what approaches can they use? The basic research plan is a designed experiment, with treatments randomly (let's assume) allocated to plots. We measure one or more responses on each plot and record context variables – measurements of things that tell us about differences between plots and farms, which might explain some of the variation in results.
Photo taken from McKnight evaluation tour in Burkina Faso, visiting project using large-N trials with farmers
Standard analysis of designed experiments uses a suite of tools starting with ANOVA, F and t-tests, tables and graphs of treatment means and use of 'mean separation' methods, perhaps with elaborations to bring in some of the context measurements. The overall structure of the analysis is determined by the design. The methods are pretty much the same as they were when first introduced, nearly 100 years ago.
The Big Data phenomenon has led to development of concepts and approaches to data analysis that are often very different from these. For example, the emphasis is on discovery of useful patterns and relationships, rather than testing hypotheses about differences. This is because with enough data, almost any standard test will give you small p-values, but that does not mean you have found out something that can be exploited. As our trials are now much larger (in terms of the numbers of repetition across farms), than when the theory and practice of analysis of experiments was developed, are there ideas for Big Data approaches that we can exploit?
I think the answer is yes, but it would be a mistake to adopt Big Data practice wholesale. For a start, our datasets coming from trials are indeed much larger than they used to be, but they are still tiny compared to those in many Big Data applications.
Diagram demonstrating Designed Experiment vs. Big Data
I find it useful to think of a spectrum of approaches; from those typical in traditional analysis of randomised trials to those typical in current Big Data. Where you should be on this spectrum will depend on many things, but from my experience with large-N trials done by small-scale farmers, I can make some suggestions. For example, the emphasis is likely to be on pattern discovery with the display of patterns more important than the p-values. But the scale of data available means that we still need to ground what we look for in the predictions of science – we simply don't have enough data to rely entirely on discovery through smart algorithms.
As far as I am aware, this is an area in which we still need rigorous, and consistent development of principles and accompanying experience leading to good-practice recommendations. When we have those, it might be easier to convince researchers that there is solid basis for doing things differently.
What are your views on different data approaches? Do you prefer traditional analysis methods for large N-trials or do you rely more on pattern discovery? And do you feel there is enough grounding in these latter principles or that they need further refining and best-practice recommendations made? Do feed back your thoughts – we look forward to hearing from you!
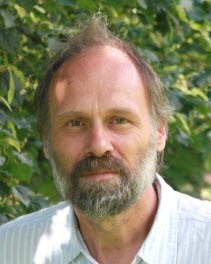
Author: Ric Coe
Ric’s main focus is on improving the quality and effectiveness of research for development using the application of statistical principles and ideas. He is particularly interested in research design, including the design of complex integrative research projects.
0 comments for "Beyond ANOVA – Big Data and Large-N trials":
Add a comment:
We run an anonymous commenting system. If you are not logged in, we do not collect any information on who you are when you leave a comment. This means we manually confirm comments before they appear on the site.
If you want to have a comment you submitted deleted, please contact us, giving the date of the comment and name of the article.