Blog
Statistics for Sustainable Development > Blog > Do the means justify the Ns? Our thoughts on sample sizes
Do the means justify the Ns? Our thoughts on sample sizes
In this week’s blog, Ric Coe and Chris Clarke explore sample sizes; the challenges around them and types of errors that occur at various stages, as well as looking at how we can look beyond and move past these. To help set the scene, here are a couple of statistical quotes to get us thinking:
“To call in the statistician after the experiment is done may be no more than asking him to perform a post-mortem examination: he may be able to say what the experiment died of” - Sir Ronald Aylmer Fisher.
“We found there was a lot of variation between the villages so in future we will work in just one of them” – Research leader at a well-known organisation.
Do either of these sound familiar to your data practise…?
The problem
Statisticians like to quote Fisher (the originator of a lot of what we use in applied statistics) on the need for researchers to consult on study design before they collect data. When we are consulted, the commonest question is What sample size do I need? It’s a reasonable question. If the sample size or number of replicates is:
- Too small, then the study will not achieve its aims and be a wasted effort.
- Too large, then the study will collect more data than needed and also be a waste.
Notice that the ‘waste’ here is not just the researcher’s time and money, but also the time of everyone taking part, and less tangible things like intrusiveness. The question needs asking for research in almost any domain, whether you are studying people, places, trees or organisations. Researchers in many areas have common practices based on rules such as ‘monitoring studies need a sample size of at least 50’, ‘take 10% of the population’ or ‘4 replicates are adequate for agronomic trials’. These are often based on a bit of wisdom that is now applied well outside its ‘use-by’ range.
So, can statisticians answer the question? Yes… and no. Yes, because there is plenty of relevant and well-developed theory and tools to put it into practice. Search for ‘sample size determination’ using Google and you will easily find a mass of resources including free, easy-to-use software. Just type in a few parameters to define the problem and out comes the answer. Easy!
Image 1: Example of an online sample size calculator. Source: https://www.surveymonkey.com/mp/sample-size-calculator/
But in practice it is often very hard to apply the theory or use the sample size tools correctly. There are two main reasons for this:
- They require information that you don’t have
- They only consider some of the factors that need to be taken into account.
Let’s look at both of these points in a bit more detail.
Information you don’t have
Using the standard formulae to estimate required sample size requires three basic bits of information:
- The exact aim or question. That is easy if it can be phrased as something like ‘estimate the average bean yield’. But most real studies have multiple aims, some of which are hard to turn into quantitative statements that can be plugged into the formulae. For example, in addition to the mean yield, we might also want to understand ‘the factors that drive variation in yield’ and find out ‘what farmers feel about current levels of production’.
- The precision you need for your answer. A common answer to that should be ‘as precise as possible’, but that is not good enough. You need to understand and put into numbers the trade-off between cost and precision.
- The amount of variation that will be found when sampling. If all the population are very similar you do not need to measure many, but if individuals are variable you will need more of them. In some situations, we might have some idea about the variation. But real problems usually require understanding variation at multiple levels – for example, between individuals in a household, between households in a village, between villages in a region, between regions - and it’s rare to have much idea about that before data has been collected.
Non-sampling error
The sample size formula you can find in books and the tools on the Internet are all based on the idea of ‘sampling error’. That is, the deviation between the true answer and your data that occurs because you only measure a sample of the population. But in any practical study there are many other sources of deviation or uncertainty in your answers; the non-sampling errors.
Image 2: Diagram showing potential sources or error. Source: https://creativemaths.net/blog/sampling-and-non-sampling-error/
There are also other factors that impinge on the sample size. Whole books on survey methods describe these, the ways they can be handled and their relationship to sample size. Here are a few general points:
- Data from real studies are subject to all sorts of influence from bias, mistakes, muddles and messes. Many of these tend to increase with the size of the study particularly for researchers and teams who are not used to the type of work. Organisations such as national statistics offices spend years of high-quality professional time working out how to minimise such problems. Researchers doing one-off studies rarely have that luxury.
- Costs have to drive a lot of the decisions. This means that the question sometimes has to be turned around to ‘how can I get the most information with the budget available?’ That is also something that can be modelled, but again will need information you don’t have – for example, ‘what is the cost of going to a new village compared with sampling more households in the first village?’
- Workflows and bottlenecks are strongly influenced by sample size. If you have a sample of 100 you might be able collect and process all the data yourself. If you have 1000 you will need a team of people. That is not just a cost in terms of payments to them. They need training and supervising. You need to develop workflow patterns and standards, quality control procedures, audit trails and reporting procedures.
So, what do we do?
As so often with statistics applied to the real world, the answer is to use the theory and principles we have, but to combine them with pragmatism. So, in practice we should use the theory as far as we can but treat the answers as guidelines rather than providing all the answers. For example, I can make some reasonable guesses and get an estimated sample size of N=450. That tells me that 45 is far too small and 4500 is probably much larger than necessary. Next think of factors that might suggest modifying the number – rules of thumb are good for this. At the same time, you should also:
- Look at what others have done in similar situations and assess whether it looked about right.
- Think of what you did last time and how it should be modified.
- Think about all the practical issues of carrying out the study and what implications they have for sample size.
Expect to iterate. That means thinking through the cycle of objectives – plan – sample size, then assessing what is feasible and going back to earlier steps. You might even have to go back to the beginning to modify the objectives and details of the design and then update the sample size ideas again. Continue until you have a sample size that is manageable and, as far as you can tell, will lead to meeting your objectives.
But whatever you do, sample size determination should be a positive and rational part of the design. You should be able to give an answer to ‘why did you choose that sample size?’ which is not simply ‘well that is what we always do’.
What are your views on sample sizes and your approach to calculating this? We’d be interested in hearing how you go about it and what approach/es have worked well in the past. And as ever, let us know if you have any questions! We look forward to hearing from you ?
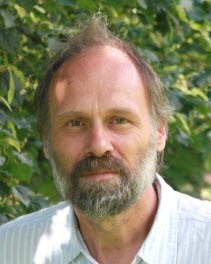
Author: Ric Coe
Ric’s main focus is on improving the quality and effectiveness of research for development using the application of statistical principles and ideas. He is particularly interested in research design, including the design of complex integrative research projects.
0 comments for "Do the means justify the Ns? Our thoughts on sample sizes":
Add a comment:
We run an anonymous commenting system. If you are not logged in, we do not collect any information on who you are when you leave a comment. This means we manually confirm comments before they appear on the site.
If you want to have a comment you submitted deleted, please contact us, giving the date of the comment and name of the article.